Learn Data Science
Data Science Lessons each contain a deep-dive into core Data Science topics, divided up into six modules. Every single lesson contains conversation "office hour"-style lectures with Professors Wade Fagen-Ulmschneider and Karle Flanagan, written explanations, example worksheets, practice questions, and more!
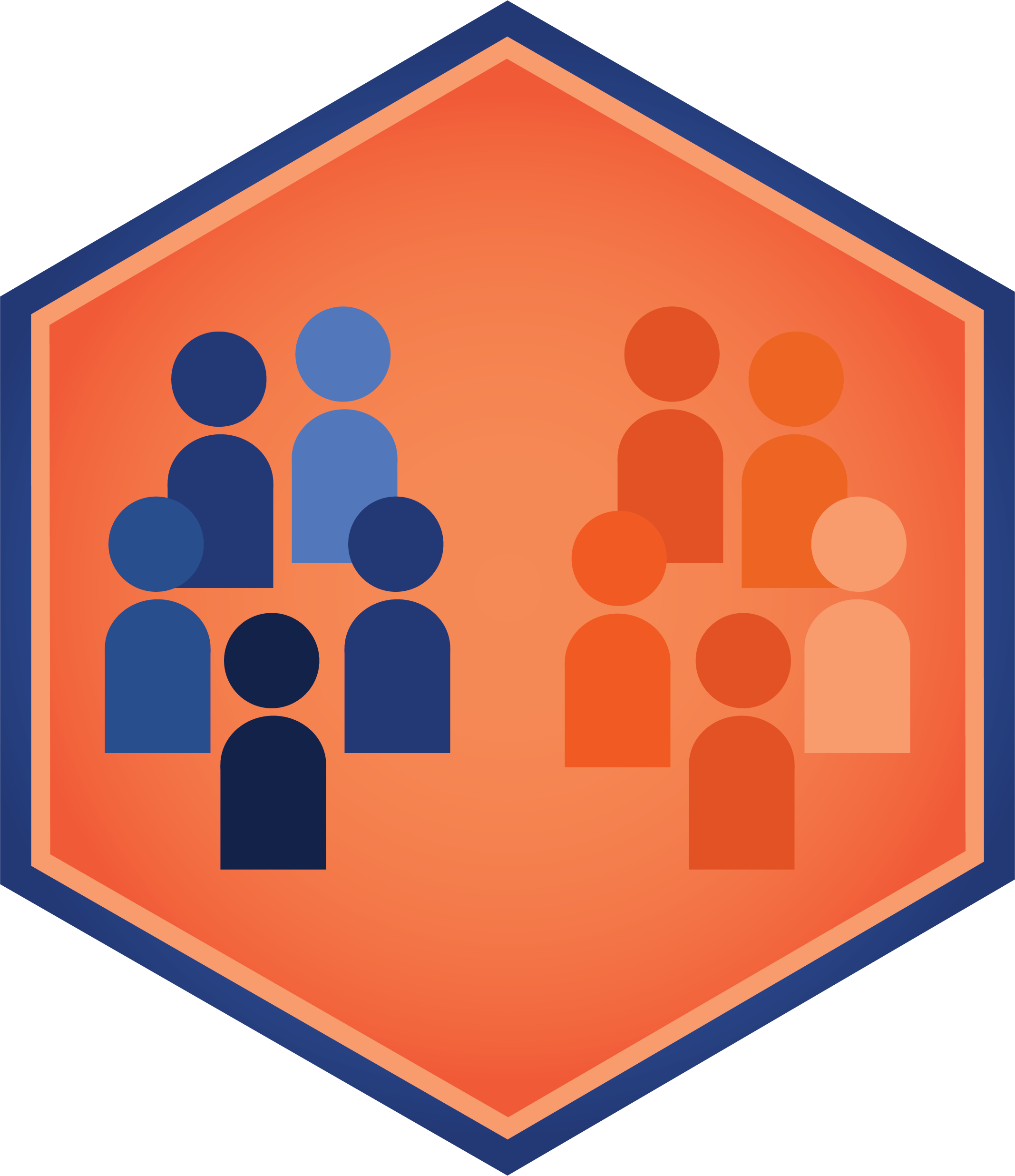
Module 1: Basics of Data Science with Python
"Basics of Data Science with Python" provides a strong introduction of the field of Data Science. You will understand best practices in designing good, great, and ideal experiments, use Python to load data into DataFrame, and manipulate DataFrames in Python to explore subsets of data.
1-00
» What is Data Science?1-01
» Types of Data1-02
» Experimental Design and Blocking1-03
» Python for Data Science: Introduction to DataFrames1-04
» Row Selection with DataFrames1-05
» Observational Studies, Confounders, and Stratification1-06
» Simpson's Paradox1-07
» DataFrames with Conditionals1-08
» Software Version Control with git
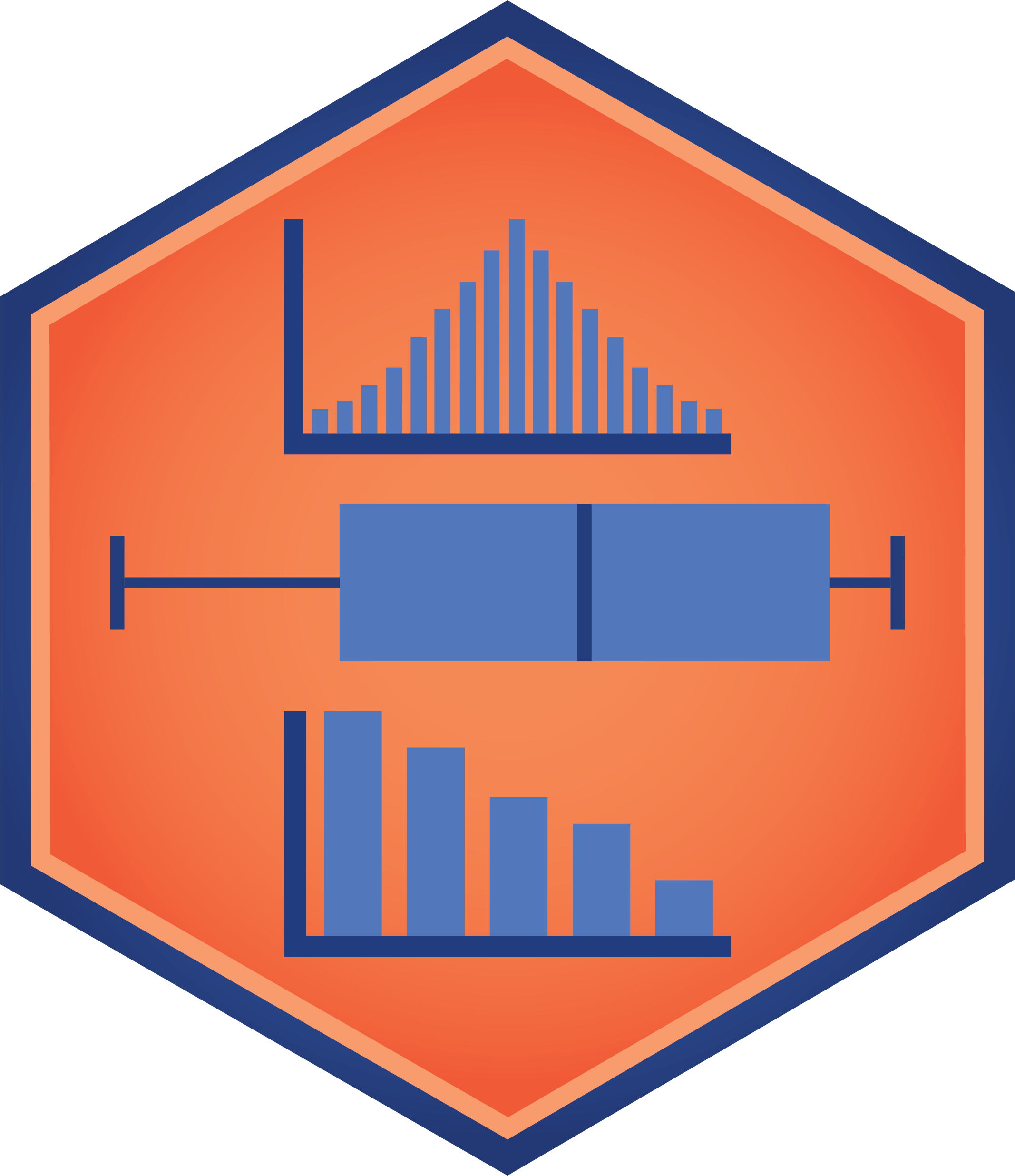
Module 2: Exploratory Data Analysis
"Exploratory Data Analysis" teaches about the tools and techniques to begin to do exploratory data analysis on real-world datasets. You will learn several methods of analyzing statistical properties of the data and how to calculate and apply these properties using Python. Finally, you will create simple data visualizations showing an overview of the data.
2-01
» Exploratory Data Analysis Overview2-02
» Descriptive Statistics2-03
» Grouping Data in Python2-04
» Histograms2-05
» Quartiles and Box Plots2-06
» Basic Data Visualization in Python
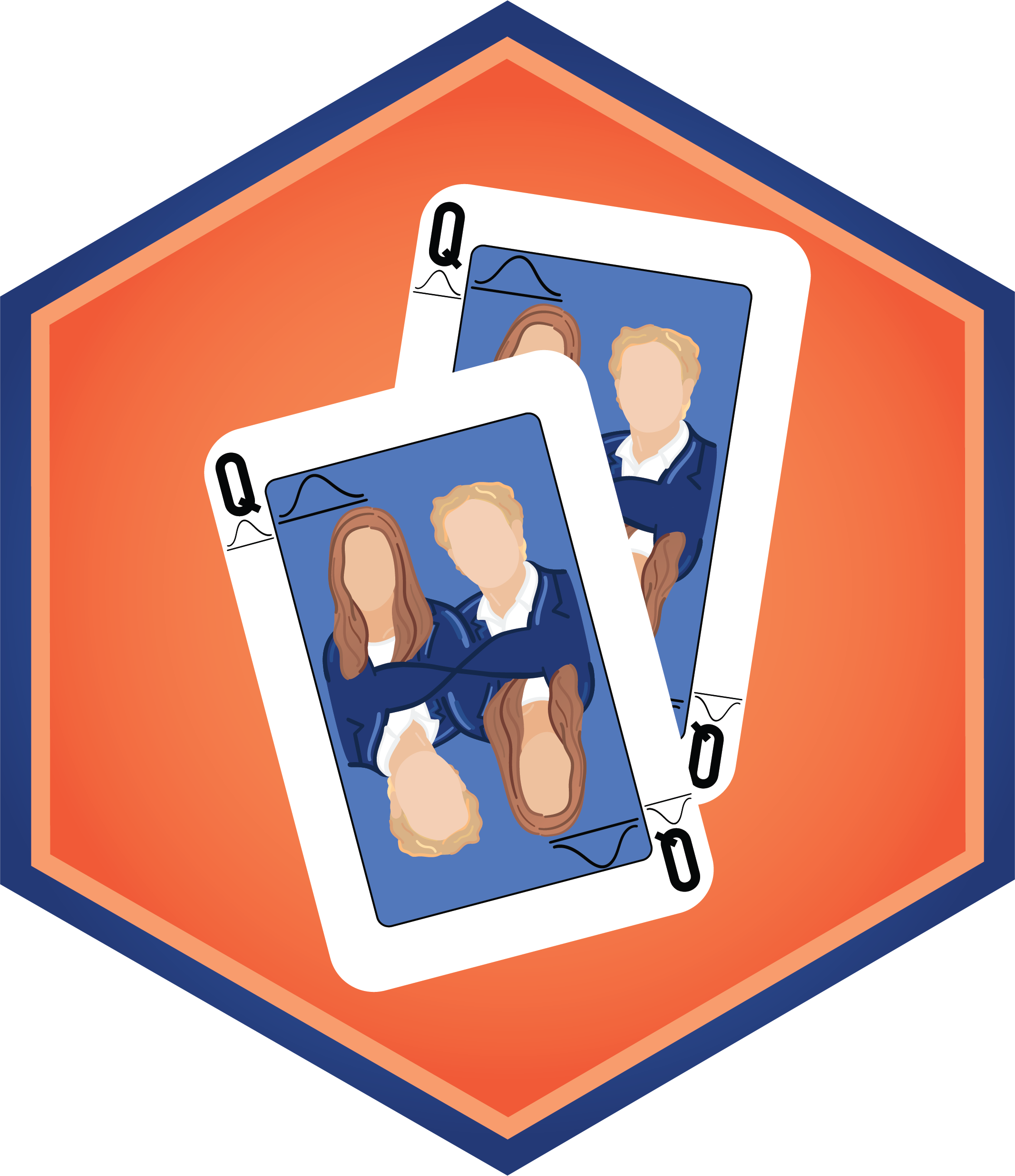
Module 3: Simulation and Distributions
"Simulation and Distributions" provides an exploration into the world of computer simulations. Beginning with simulating simple events, like rolling a dice where the expected outcome is known, you gradually build increasingly complex simulations. You will find many simulations result in common distributions, such as the Normal Distribution, which you will learn has many interesting properties all its own.
3-01
» Overview of Simulation3-02
» Random Numbers in Python3-03
» For-Loops in Python3-04
» Simple Simulations in Python3-05
» Sample Space3-06
» Conditionals in Python3-07
» Functions in Python3-08
» Normal Distribution3-09
» Law of Large Numbers
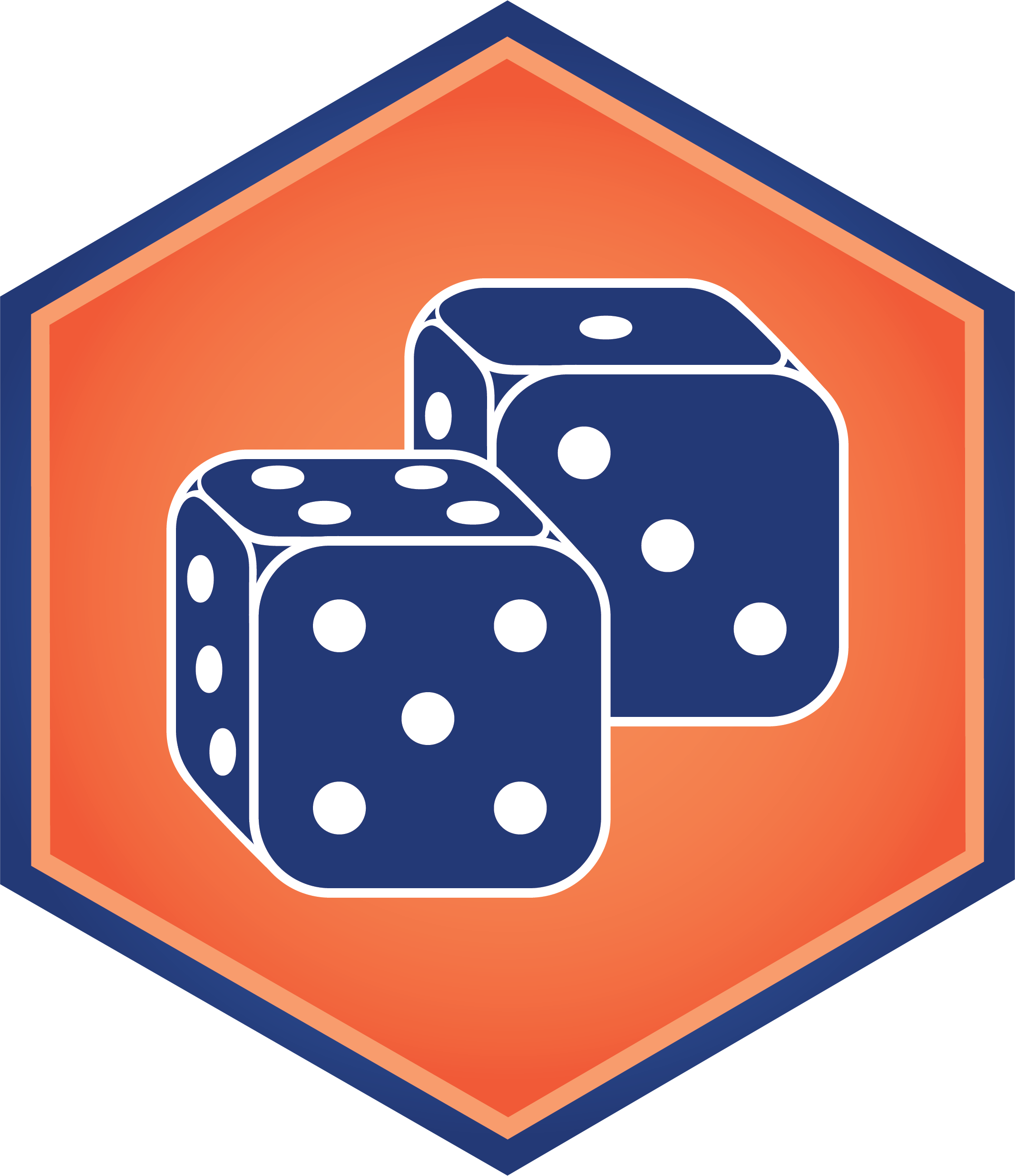
Module 4: Prediction and Probability
"Prediction and Probability" begins with a deep-dive into probability and using probabilities to make informed predictions on future events. You will complete dozens of problems on basic probability, explore how to describe dependent probabilistic events, and use Python to make predictions under uncertainty.
4-01
» Probability Introduction4-02
» The Monty Hall Problem4-03
» Multi-event Probability: Multiplication Rule4-04
» Multi-event Probability: Addition Rule4-05
» Conditional Probability4-06
» Bayes' Theorem
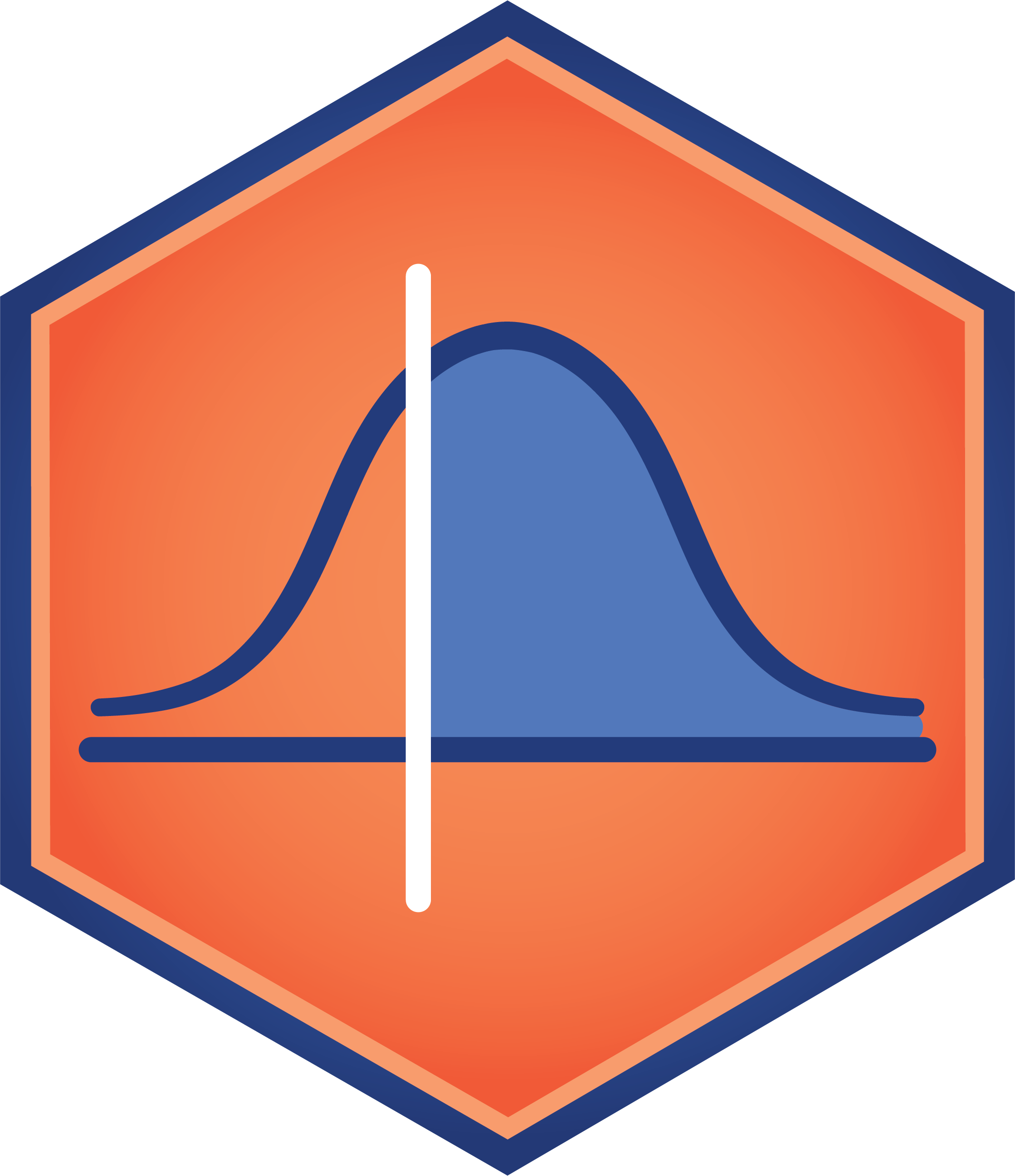
Module 5: Polling, Confidence Intervals, and the Normal Distribution
"Polling, Confidence Intervals, and the Normal Distribution" starts with an exploration of different sampling techniques. You will learn how bias and sampling variability can affect the results of surveys. From that, you know how to use expectation and inference as a way to make predictions and decisions under uncertainty.
5-01
» Random Variables5-02
» Bernoulli & Binomial Random Variables5-03
» Python Functions for Random Distributions5-04
» Central Limit Theorem5-05
» Polling and Sampling5-06
» Confidence Intervals5-07
» Hypothesis Testing
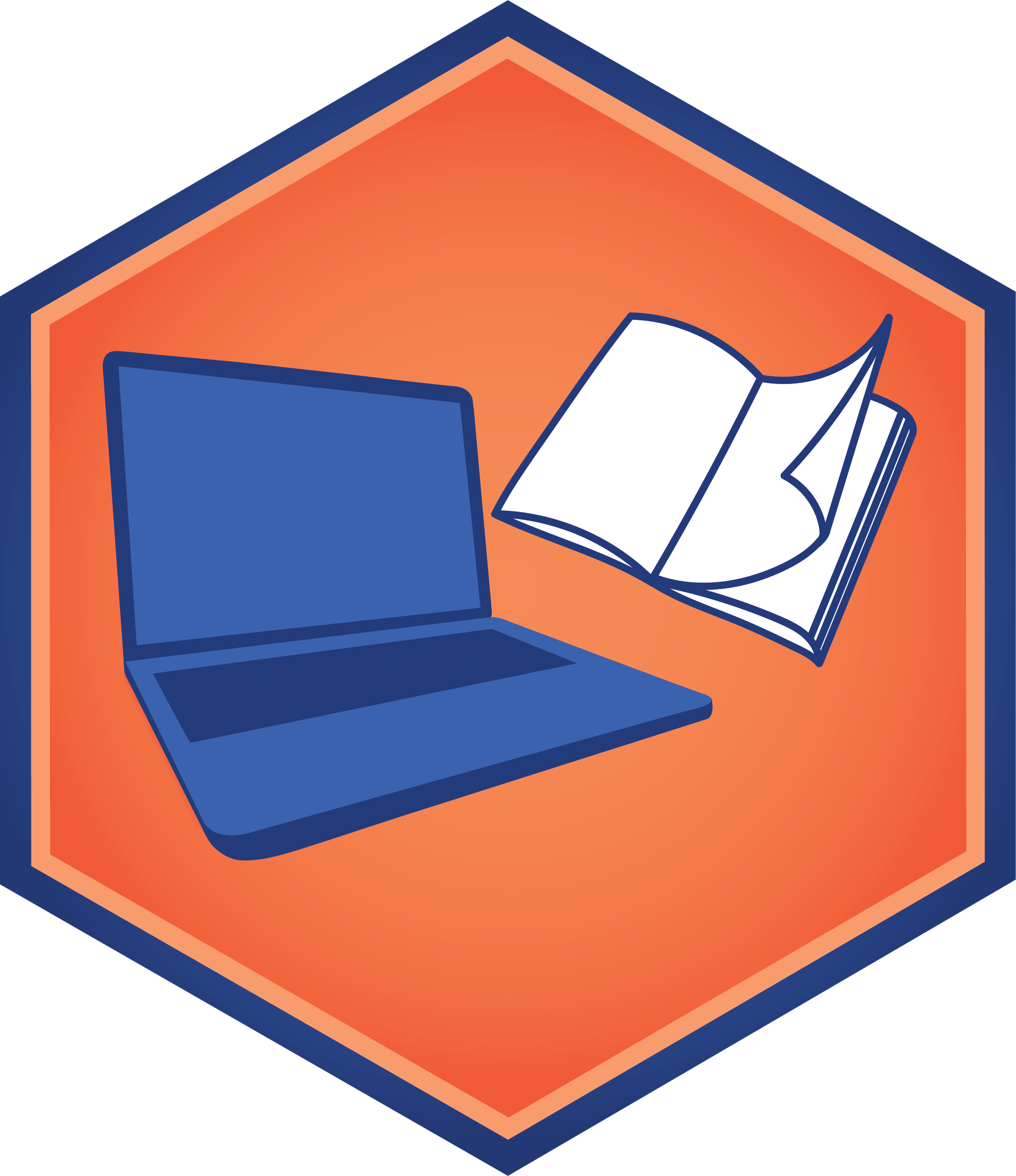
Module 6: Towards Machine Learning
"Towards Machine Learning" applies all of the foundational knowledge applied in the previous modules to using modern techniques to help computers discover common similarities in data and to predict future outcomes based on previously-seen events. Completion of this and all other modules provides you with the ability to advance to dedicated machine learning courses.
6-01
» Overview of Machine Learning6-02
» Correlation6-03
» Linear Regression6-04
» Machine Learning Models in Python with sk-learn6-05
» Clustering6-06
» Towards Machine Learning in Python